22 December 2023
The impressive capabilities of AI have captured the world’s attention, leading many to imagine—with excitement or trepidation—the kind of future this “artificial intelligence” will bring. As with any potentially transformative technology, however, the impact of AI on the world will ultimately result from decisions made by humans. Through action or inaction, it is people, not machines, who will determine what tomorrow’s society will look like. When it comes to the economic implications of AI, there is particular concern about how it could be used to disrupt labour markets, eliminate jobs and increase inequality. While current trends in automation make these outcomes disturbingly plausible, they are not inevitable.
At this critical moment, we have a unique opportunity to choose a different path, guiding the trajectory of AI in a way that empowers workers. Of particular importance is a topic that has received little popular attention: the choices we make about how AI is developed. Decisions made during the development process reflect society’s values and, in turn, shape the values that are embedded in resulting AI models. The process thus represents a key point of intervention for creating AI that benefits everyone, including workers.
Paradoxically, the sensationalized narrative around building technology that is potentially capable of human-like reasoning sometimes glosses over the fact that human intelligence and reason are central to training, building and maintaining useful AI models. These systems are meant to emulate our behaviour and decision-making, but the ability of AI to mimic humans is only possible because the model learns from humans. This happens during the development of an AI model, when human judgment, opinions and activity are captured in the form of data. Though the Internet has generated a lot of data related to human and social activity, that data is not categorized and structured in a way that makes it suitable for training AI models. To fill the gap, millions of people around the world, known as “data enrichment workers”, have been recruited to categorize, label, annotate, enrich and validate the datasets upon which AI models are built.
The AI industry must shift its focus from “getting access to data” to creating datasets, which requires labour.
Data enrichment workers perform a wide variety of activities, such as labelling radiology images to build AI cancer-detection models; labelling toxic and inappropriate posts online to build content moderation algorithms or make the outputs of large language models less toxic; labelling videos captured from people driving to train “self-driving” vehicles; editing outputs from large language models to improve their usability; and much more. This massive, global, collective effort to train AI results in models that represent the collective human intelligence of all those who have contributed their judgment in the form of data. For users, the true value of AI is the access it provides to this great repository of human intelligence, which can be used to help us make decisions and solve problems.
Yet the central role human contributors play in enabling high-quality AI models is at odds with how these workers are treated and compensated. Rather than celebrating and recognizing the critical importance of human intelligence in fuelling the AI advances that have captured our imagination, data enrichment work remains undervalued, underpaid and underappreciated. Consistent with broader outsourcing trends, much of this work is done in low-income countries in the Global South, where lower wages can be paid. In addition to low pay and wage uncertainty, data enrichment workers face a lack of benefits, psychological harm from reviewing toxic content, lack of power to contest their conditions, unpredictable streams of work, high transaction costs for equipment and other forms of support to enable their work, and overall precarious conditions. While we can see that the poor conditions these workers are subjected to are aligned with broader, negative historical and economic trends, they are not inherent characteristics of the work itself and can be changed.
Part of the difficulty in improving conditions for data workers is the need for more widespread acknowledgement that data work is, in fact, work. Many early AI advances came from accessing and using data generated by commonplace user activity on the Internet. As the industry tries to build higher quality AI models, aspiring to both human-like reasoning and human-level creativity, we have seen and will continue to see greater demand for higher quality datasets. The AI industry must shift its focus from “getting access to data” to creating datasets, which requires labour. More artists, writers and people with specialized knowledge are being enlisted to help create more specialized AI models. As these more advanced models are built and become widely available, we are provided with exciting opportunities to access, learn from and utilize others’ expertise, as captured by AI.
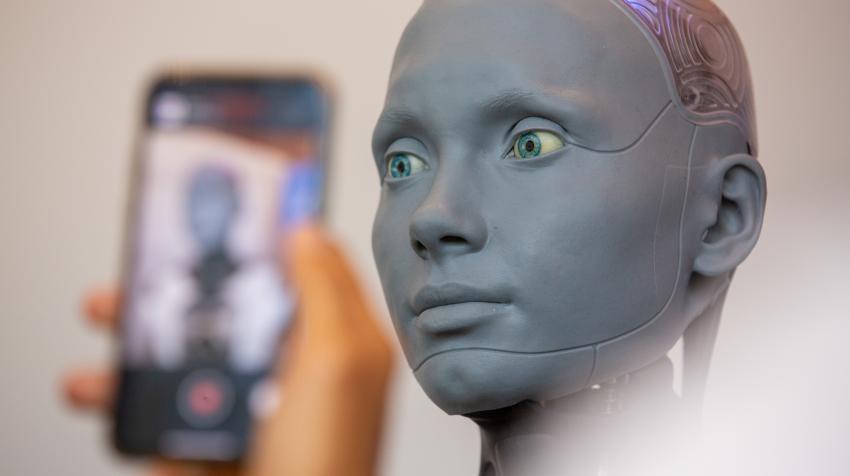
As human contributions drive the growth of the AI industry, we must adapt our understanding of what constitutes work in the AI economy and how different types of labour generate value and should be valued. If we build an AI ecosystem that appropriately values these human contributions, we have the opportunity to build a more equitable economy in which more people benefit from AI advances. While AI certainly has the potential to transform the global economy, we have the power to design an economy that will enable AI development to better serve society’s interests.
To create the means to reimagine the AI economy and steward AI development to positive outcomes for people and the planet, we must increase our scrutiny over how AI is developed and target interventions accordingly. Currently, limited concern is shown for data enrichment workers. Recognizing the intense amount of human labour necessary to build AI would overshadow more electrifying narratives about building machines capable of human-like thought. Greater emphasis has been placed on the outcomes of AI deployment, as opposed to a more mundane, intentional analysis of our approach to AI development. This focus on deployment may contribute to our tendency to overlook the people behind data enrichment. This oversight has resulted in a global data supply chain that is haphazard, disjointed and opaque, and which takes data enrichment workers for granted. Even critical discussions on mitigating the potential negative social and economic impacts of AI sidestep the issues surrounding the creation of AI tools.
To build a more equitable economy and society around AI, policymakers, civil society advocates, journalists, industry practitioners and other key stakeholders should focus on interventions that target the development process and help ensure that the benefits resulting from this technology are equitably distributed. While governments and industry recognize the productive potential of AI to generate economic gains, they should also work to avoid exacerbating inequality in the AI economy.
When examining the AI development process, it is obvious that data enrichment workers and other types of creators contributing their intelligence are the foundation of this technology. At the societal level, we should create an economic framework that appropriately values these contributions, so that those who are helping enable economic gains from AI are also benefiting from them. Furthermore, focusing on the development process can help push us to develop a better understanding of the conditions under which humans are contributing to AI datasets so we can have a better understanding of the information empowering these tools. This is important for ensuring not only that these workers have protections, but also that the resulting AI models are safe and reliable enough for humans to use in the real world. It would allow us to think more intentionally about how we encode values, beliefs and our collective understanding of the world into the AI models that we intend to use every day.
The UN Chronicle is not an official record. It is privileged to host senior United Nations officials as well as distinguished contributors from outside the United Nations system whose views are not necessarily those of the United Nations. Similarly, the boundaries and names shown, and the designations used, in maps or articles do not necessarily imply endorsement or acceptance by the United Nations.